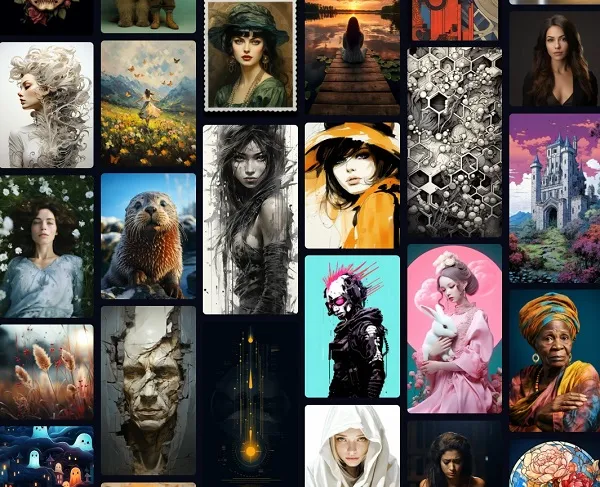
Models or algorithms that generate novel output like text, photographs, movies, code, data, or 3D renderings from massive amounts of data they are trained on are known as generative artificial intelligence (AI) or GenAI. The models 'create' fresh material by making predictions based on past training data. Recent developments in the field may cause us to rethink our strategies for making media. The field of machine learning encompasses generative AI systems. There are several real-world applications for generative AI, such as the development of novel product designs and the enhancement of operational efficiency. These widely used GenAI technologies have caused a digital society-wide earthquake, transforming thousands of people into digital artists overnight. This exciting area of AI has the potential to greatly improve creativity in many fields, and perhaps even make it automatic.
Generative artificial intelligence (AI) can be traced back to the early days of AI research in the 1950s and 1960s. Back then, AI scientists were working on rule-based systems to mimic human cognition and decision-making. Early models of computing suggested by IT pioneers like Alan Turing and John McCarthy were crucial in building the groundwork for GenAI since they were founded on the assumption that machines could one day replicate human intelligence. Neural networks are able to forecast and make judgments without being explicitly programmed because they have been trained to recognize patterns in datasets. GenAI's innovative prowess stems from a specialized neural network dubbed a Generative Adversarial Network (GAN), which was created in 2014 by Ian Goodfellow and his colleagues. To increase the quality of generated data, GANs incorporate two neural networks into the design, a generator and a discriminator, which compete with one another. Generative models were first employed for speech recognition, image processing, and natural language processing (NLP), and they quickly became the subject of experimentation. In 2014, with the advent of generative adversarial networks (GANs), a class of machine learning algorithm, generative AI applications were able to generate convincing human-like visuals and audio.
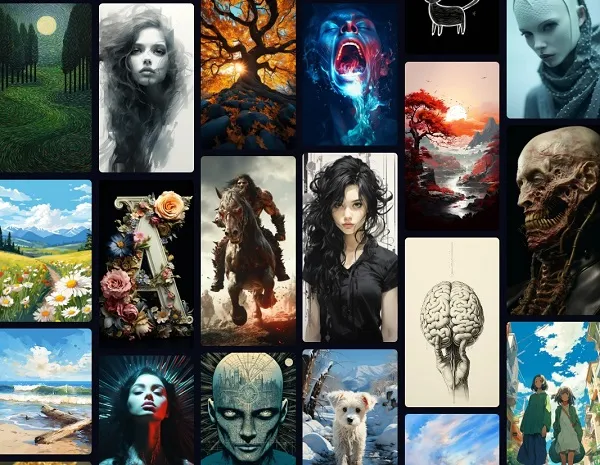
The discipline of data analysis has been revolutionized by generative AI. Generative AI is able to do things that would be extremely challenging, if not impossible, for a human to do on their own: analyse massive volumes of data in real time and detect patterns to make predictions. This has greatly improved the effectiveness and precision of industries including banking, advertising, and medicine. Generative artificial intelligence (AI) picture style transfer technologies like DeepArt and DeepDream have proven their worth by empowering people to make extraordinary works of art from seemingly mundane photographs. To generate an answer to a given query, generative AI models sift through massive amounts of data collected from around the web and utilize the knowledge they've gained to make educated guesses. These forecasts are based on the information the models are given, but their accuracy is not guaranteed. Deep learning, a computationally intensive process, is used by generative AI models to identify and exploit patterns and structures present in big data sets in order to generate novel, plausible results.
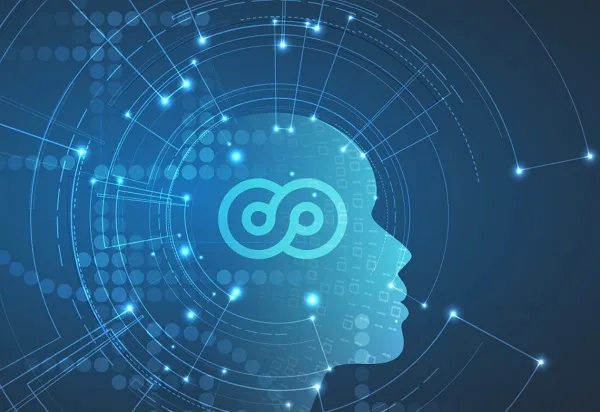
Examples of generative AI:
In 2023, generative AI became wildly popular. The ChatGPT and DALL-E programs developed by OpenAI are excellent examples.
ChatGPT: It is a chatbot powered by AI that has been trained to engage in conversation with humans in a way that is both natural and conversational. A web-based, free version of ChatGPT was made available in November 2022. It can learn from queries asked in text and respond intelligently.
DALL-E / DALL-E 2: OpenAI with the ability to interpret textual input and generate visual content.
Google Bard: It's Google's answer to ChatGPT, a generative AI chatbot. Based on Google's massive LaMDA (Language Model for Dialogue Applications), Bard is a text-to-text generative artificial intelligence interface. Like ChatGPT, Bard is an AI-driven chatbot that can respond to queries and generate new text in response to user input.
Midjourney: This next-gen artificial intelligence model, developed by the San Francisco firm Midjourney Inc., takes textual cues and turns them into visual outputs that are reminiscent of DALL-E 2.
GitHub Copilot: Artificial intelligence-driven code completion suggestions for VS Code, Neovim, and JetBrains IDE.
Llama 2: Create conversational AI models for chatbots and virtual assistants with the help of Meta's open-source big language model, which is comparable to GPT-4.
xAI (Explainable AI): In July of 2023, Elon Musk unveiled a new generative AI firm after having previously funded OpenAI.
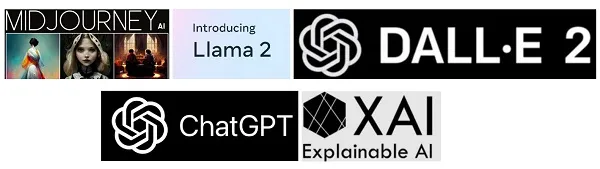
Generative AI Models:
Transformer-based models: In order to comprehend the connections between sequential data, such as words and sentences, transformer-based models are trained on massive datasets.
Generative adversarial networks (GANs): Two neural networks, a "generator" and a "discriminator," work in opposition to one another to simulate the appearance of real data. The discriminator checks the legitimacy of the data produced by the generator. A game is played between the generator and the discriminator, with the generator's goal being to produce data that the discriminator cannot tell apart from the genuine data, and the discriminator's goal being to improve its ability to identify the fake data. They utilize an architecture that takes the complete input text into account to produce writing that is both logically sound and well-suited to its setting.
Variational autoencoders (VAEs): VAEs use two networks, an encoder and a decoder, to analyze and create data. The encoder receives data and transforms it into a more compact and easily processed format. The decoder then takes the compressed data and reconstructs it into a form that is similar to but distinct from the original data. They generate new information by first encoding it into a latent space (a compressed representation of the information) and then decoding it. VAEs are able to provide unique data instances that share similarities by incorporating a randomization factor into the encoding process.
Multimodal models: Complex results can be generated by multimodal models because of their ability to comprehend and interpret numerous modalities of information at once, including text, images, and audio.
Generative AI impacts:
- Data Analysis
- Language Translation
- Customer Service
- Education
- Digital Marketing
- Creativity
- Healthcare
- Finance
- Environmental Science
- Creating
- Discovering
- Summarizing
- Automating
Limitation/Disadvantages of GenAI:
The risk of disseminating false information and malicious material is a key issue with generative AI systems. Inappropriate usage or administration of generative AI could compromise national security.
- Hallucination (Make up things that don't make sense or are contradictory to reality or common sense.)
- Difficulty with content moderation
- Ethical issues
- Legal and regulatory issues
- Copyright issues
- Privacy issues
- Autonomy and responsibility
- Political implications
- Energy consumption
- Cybersecurity and fraud
- Intellectual property (IP) and copyright
- Lack of transparency